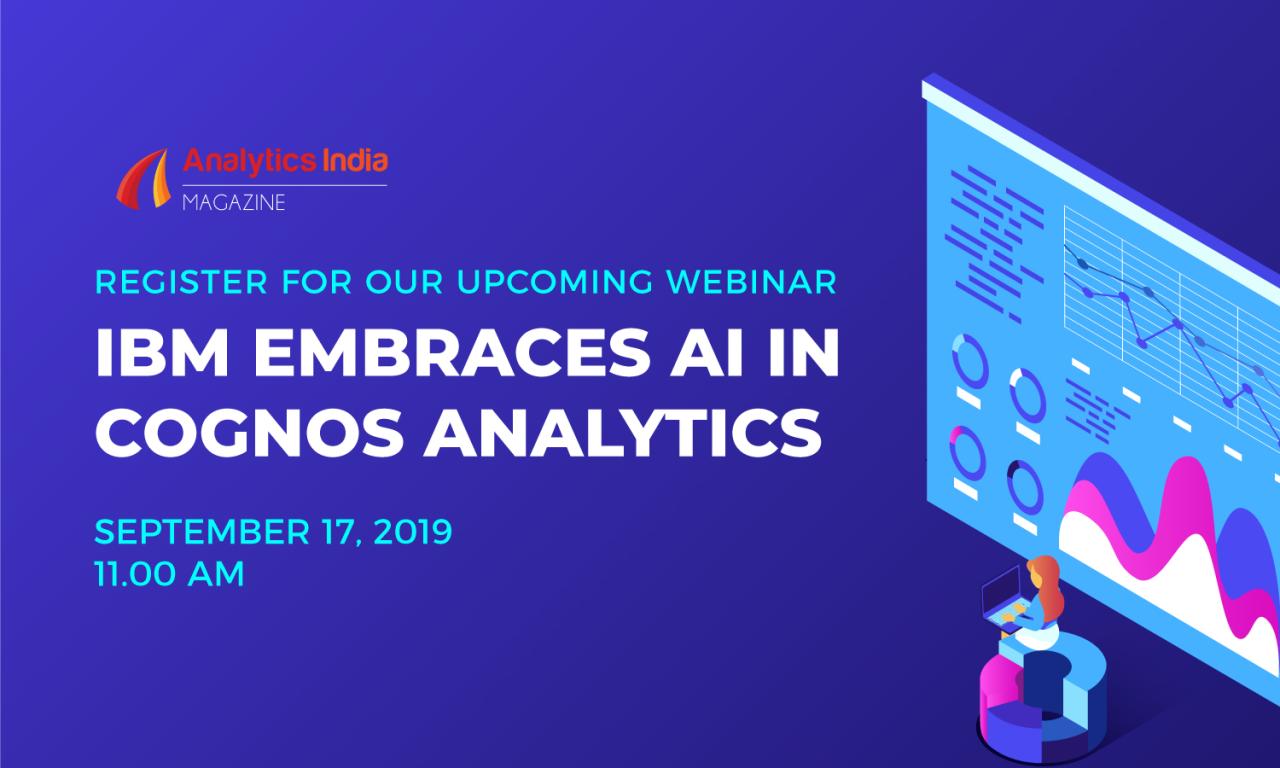
Natural language processing (NLP), business intelligence (BI), and analytics have all evolved in parallel in recent years. NLP has shown the ability to make business intelligence data more accessible. But there is still a lot of work to be done to adapt NLP for use in this highly competitive environment.
Integrated NLP-enabled chatbots have become part of many business intelligence-based systems with search and query capabilities. Old and emerging players in the field of business intelligence are competing fiercely as data science and technology share similar goals. But competition has spurred innovation.
Domo, Google Looker, Microsoft Power BI, Qlik Insight Advisor Chat and Tableau, SiSense Fusion, and ThoughtSpot Everywhere have all received NLP updates. This has made data consumption more convenient as business users retrieve data using natural language queries.
Create a world for ChatGPT
More innovation in a wide range of products. Like other areas of technology, it is changing dramatically as major language paradigms such as ChatGPT from OpenAI come online.
Signs of improvement in the ChatGPT NLP experience came last month when Microsoft announced that Power BI development experiences based on this model would be delivered through Azure OpenAI services. This week, the company introduced AI capabilities to manage virtual agents.
Also this week, SalesForce announced an OpenAI integration that combines ChatGPT for Enterprise with SalesForce's AI model for a variety of tools, including automatic summarization that can impact business intelligence workflows.
Wake up from stress
“Natural language queries and natural language interpretations are very common across all BI analytics products today,” Constellation Research analyst Doug Henchen told VentureBeat. But he said the road has been difficult at times.
When NLP improvements were first introduced to business intelligence systems, "there was some fanfare," says Henschen. Enterprise developers must work to create a common language in the domain in which data users reside. It involves identifying synonyms that people can use to describe the same topic. He says educational tools and behind-the-scenes tools are improving the automation of settings.
"For the most part, BI products handle it better," Henson says. "Now we have a new wave of large language models and generative AI… another level of technology."
Advanced Business Intelligence NLP
In most business intelligence systems, data is collected in the traditional way: access the application, generate the necessary reports, and filter the information using dashboards. But this often lengthy process requires some technical knowledge. This means lower acceptance rates.
This is why companies often use data scientists and data analysts to extract information from their business intelligence systems. But managers want broader acceptance in organisations. An increasing number of global organizations are using NLP-based business intelligence chatbots that can understand natural language and perform complex business intelligence tasks.
“Business intelligence moves from reporting to anticipating and ordering appropriate actions based on real-time data,” said Sarah O’Brien, vice president of cross-market analytics at ServiceNow.
“These activities can now be embedded in spoken language and drawn from a variety of sources, as a result of the explosion of innovation in natural language processing,” says O'Brien. "Business intelligence provides the context, while NLP provides the content."
"Today's chatbots can efficiently extract data from multiple sources such as lobbying and customer relationship management systems and integrate with many third-party messaging applications such as Skype for Business and Slack," said Vaidya, Research Director at Tableau.Setlur.
“Using an NLP-enabled chatbot and a question-and-answer interface, visual analytics workflows are no longer tied to traditional dashboard interfaces. People can ask questions on Slack to quickly gain insight into the data,” Setlur tells VentureBeat.
This means that users can access important information at any time through a conversational interface without logging into the BI application. Setlur believes this has changed the way organizations think about growing their business and the type of talent they hire.
“The NLP-based analytics experience has democratized how people analyze data and gather information without having to use complex analytics tools or create complex data queries,” Setlur added.
This convenience plays an important role in shaping the organizational culture of analytics. By applying NLP to business intelligence tools, even non-technical workers can analyze the data themselves, rather than relying on IT professionals to generate complex reports.
Using NLP allows people without complex analytical skills to ask simple language questions about their data. Because people can quickly get answers to questions from complex databases and large databases, Setlur said, organizations can make better data-driven decisions.
He added that Natural Language Text and Voice Interfaces (NLI) can interpret these questions and provide intelligent answers about relevant information and inferences.
Similarly, Ivelis Rocha Bernardo, head of data and applied research at virtual reality platform Mesmerise, believes that this implementation has made data analysis more transparent and helped democratize organizational data.
“Stakeholders and leaders can request data with questions, and their core business intelligence platforms can respond by providing relevant graphics. This is the next level of data analytics, opening up business intelligence and analytics where teams can focus on more detailed tracking and indirect data. Bernardo told VentureBeat about it.
Automate your business intelligence workflow with NLP
Organizations can automate many workflows by using natural language processing to find the information they need.
"Search engines can use NLP algorithms to suggest relevant results based on users' past search history and interests," said Setlur of Tableau VentureBeat. These search engines are getting more and more sophisticated, answering onboarding questions like, “What are the terms of the trip?” or "What's the Golden State Warriors' current score?"
Predictive text generation and autocomplete are used in everything from cell phones to document creation and email. Algorithms can suggest words and phrases that match the tone of the message.
Domain must be specified
According to Bernardo Messert, collaboration is essential in the business intelligence process. He said that the implementation of the NLP model is a collaboration between groups. It is critical to get support from subject matter experts to set up the workflow architecture and work closely with the data science team.
“There are many successful [use] cases where NLP is used to streamline workflows, and one of them is social media analysis of trend or brand engagement. Another success story is chatbots that improve customer service by automating the process Answering Frequently Asked Questions According to Bernardo, they prevent you from concentrating on tasks that require interaction.
As an experienced data scientist, Bernardo advises that the best way to implement an NLP solution like this is to work incrementally, in small batches, and measure and track results very realistically.
“To effectively implement this solution, I suggest starting by identifying the use cases that the organization wants to improve. Then set long-term and short-term goals. Short-term goals should be results-related and linked to a specific project. Finally, the team will develop a long-term plan at the end of each phase. Bernardo said It needs to be re-evaluated and re-evaluated.
Also, one of the best practices for implementing NLP solutions is to focus on a specific area. "The broader the scope of the model, the greater the likelihood that the NLP model will give inaccurate results."
Problems implementing NLP in BI today
One of the main challenges in implementing NLP in business intelligence is that NLP models can be biased towards certain groups or demographics. Second, while natural language processing systems require large amounts of data to operate, the collection and use of this data can pose significant privacy risks.
“We need to focus on creating a fair and unbiased model. Before storing any data, organizations should consider the interests of users, why data is needed, and implement rules and best practices to protect user data.
NLP models can also be more complex and it can be difficult to understand how they arrive at certain solutions. Therefore, it is very important to focus on explaining the model, that is, on making it easier to understand how the model makes certain decisions.
“Computer systems must be able to decipher and interpret the many ways people request information, including in domain-specific terms (such as the medical industry). Developing robust and reliable tools to help business intelligence organizations analyze and gather information while maintaining security remains a challenge. A challenge that needs more improvement in this area.
What's next for NLP in BI?
Although NLP has developed and can help solve a number of problems, the language itself is still complex and ambiguous.
According to Yashar Behzadi, CEO and founder of Synthesis AI, the generative AI approach to NLP is still new and many developers understand how to properly create and modify models.
Naive use of this approach can lead to bias and false conclusions. However, there are startups and well-known companies that are creating enterprise versions of these systems to facilitate the development of customized models that avoid existing problems.
Behzadi predicts that in the coming years, enterprise solutions will enable companies to apply large language models to their data. In addition, model tracking and feedback solutions will become popular for real-world performance evaluation and further refinement of core models, he said.
“Traditional business intelligence needs to be supplemented, not replaced by new NLP approaches over the next few years. Technology is evolving rapidly, but business-centric solutions need to be based on proven business intelligence approaches so that there is confidence in the new approaches,” Behzadi added. .
Meanwhile, Yaniv Machover, CEO and founder of AI-powered copywriting platform Anyword, said his company sees growing demand for “copy analytics,” a business intelligence approach to managing communications with marketers across multiple channels. Makeover says we'll see BI integration with generative AI in the near future.
“With the advent of LLM, NLP algorithms can accurately summarize user-generated content without creating an endless stream of examples instead of copying them verbatim. This makes the survey summary more compelling,” Macover says.
Understanding end user preferences and needs is always imperative in natural language processing and business intelligence, as is the need to sift through large amounts of data programmatically.
It is important to note that an LLM such as ChatGPT can help overcome hurdles from a BI developer.
Such generative AI can help not only in business, but also in programming languages, says Doug Hans.
"As the next generation of natural languages, generative AI also generates code," he said. "She's big".
But he did point to a warning he called "man on the alert".
“There are a lot of stories and examples of someone trying to do something with a form and it ended up being a bad thing. So the more contexts a developer creates, the more reliable the results will be.”
Henschen companies still need human oversight and control. But he said models like ChatGPT "promise to save you a lot of time and help you build code that's as close to your needs as possible."
But you have to make sure it's correct.
VentureBeat's mission is to be the digital city arena for technology decision-makers to gain insight into enterprise technology and deals. Get our brief.
Wow, superb weblog format! How lengthy have you been running a
blog for? you make running a blog glance easy. The whole look of your website is
fantastic, as well as the content material!