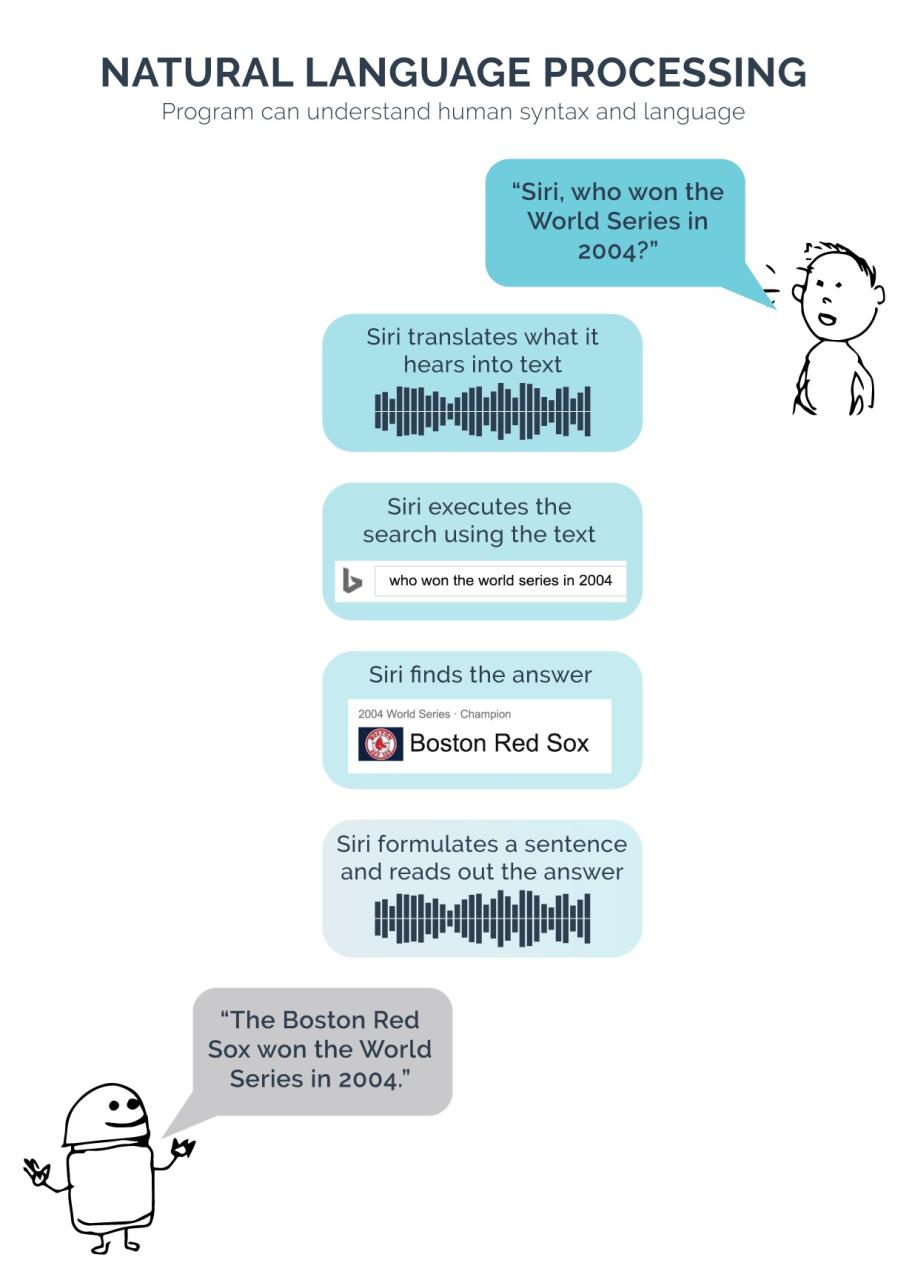
94% of all business leaders on the planet believe that AI will be critical to success in the next five years. However, according to Deloitte’s latest State of AI survey, many companies are still not realizing the value they envisioned, with 29% of respondents labeling themselves as AI “laggards” this year compared to the previous year. . last year.
According to a Deloitte survey, challenges that will limit the impact of AI include increased cost to the business and lack of full commitment from management. Industry leaders and observers in the construction industry agree that organizational challenges, not technical ones, are holding back growth.
The main takeaway is that AI should serve customers and help businesses put customers first.
Most AI projects fail “because they don’t consider the benefits of making accurate predictions, the cost of incorrect predictions, or business realities like constraints like large marketing budgets,” says Arijit Sengupta, CEO and founder of Capability. “If your AI project looks like a lab experiment and your experts talk about things like lost logs instead of profits, gains, and costs, your AI will surely fail.”
The key is to ensure decision makers are more comfortable and knowledgeable about AI, build organizational support for AI, and focus precisely on how AI can help customers. “Organizations are more likely to adopt an AI-powered approach when they are directly connected to customer value,” said Bumi CTO Rajesh Raheja. “For example, a recommendation engine that can show you next steps in a business process based on proven best practices will be more useful to a business than an engine that can show you additional products that the business can buy. Both require complex AI models. But the former clearly creates value for customers.”
We have the tools, we just don’t know how to use them. “Implementing AI depends on the value and return on investment it creates, along with the model training effort, it requires the right resources and skills to build a strong data pipeline,” said Raheja. “The results of machine learning will change with data, algorithms and evolution. The fear of unknown decisions leading to unexpected liabilities is another reason for large companies to be cautious.”
For example, Raheja adds, “AI-based loan applications are built into the data and models with inherent errors or biases that will be rejected by certain segments of the population after model refinement.
Much of this has to do with the timeliness of AI data. “AI is really a perishable commodity,” says Sengupta. “If your AI takes months to build, is trained on months of data, and the world has changed in that time, AI is no longer relevant. You should be building and deploying AI in days, not months. If you want value, repeat as the world changes.”
There are many innovative business cases that can support the adoption of AI. Examples Raheya offers include using AI to “analyze customer data entered into the system for format and semantic validation. Natural language processing and automated chatbots are other use cases for customer relationship management.
“The implementation of AI is limited by the availability of data specialists and we cannot learn how to fix this,” Sengupta added. We’re trying to teach business users to talk about AI instead of teaching AI to talk about business. Before sending a business user off to learn Python, stop and ask, “Why can’t AI understand my business needs and automatically generate Python code?” The Internet revolution didn’t happen because everyone learned to code to connect to the World Wide Web; Because almost anyone can use the Netscape browser.